AI in health: Mirage, miracle or management tool?
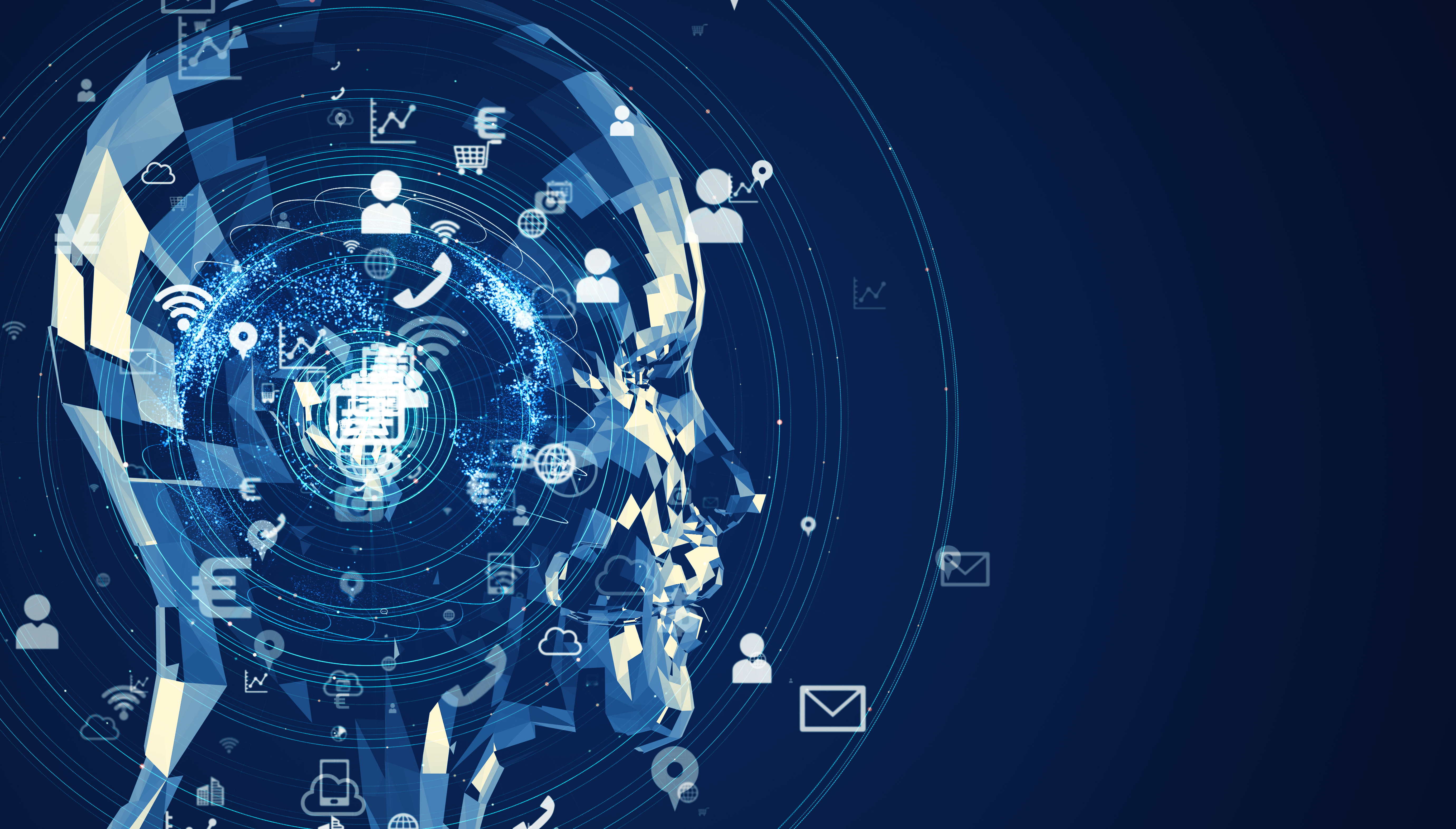
None of us know how artificial intelligence (AI) will change healthcare, but having experienced the earlier fibre optic revolution, I suspect we are in for some surprises.
On the first Monday of 1985, I walked into a corporate research centre having just finished my doctoral research on Christmas Eve. We all believed that fibre optics would mean everyone would soon feast on limitless information brought to them by invisible light sandwiched between glass. Academic and industrial labs overflowed with photonics researchers and a nearby site had so much new kit that it piled up in the corridors waiting for installation.
Conferences were packed and a bewildered waiter at one resort asked why everyone sat in the dark all day instead of playing golf.
Eventually a few things became clear. Firstly, that to transform services, any new technology must connect to the old. That means two things: it must at least answer the same questions that old technology answered and it must be able to plug into the same hardware. In the 1980s and 1990s, coaxial cable ruled and we couldn’t plug into that, nor could we answer the questions coaxial cable had already answered. Beyond that, who needed bandwidth when movies came through the letterbox, music was live, or on vinyl, and your friends had to be working or at home to take your call?
Most importantly we realised that any new technology must pay for itself, which means you need a business model. Most of our patents expired before they hit the market because nobody knew how to pay for fibre networks, or what to expect in return. Eventually, Steve Jobs and others cracked the bandwidth conundrum.
These three key questions probably apply to AI in healthcare too:
- Can it answer the same questions that other approaches already answer, but better and more cheaply?
- Can it plug into what is already in use?
- Can it deliver new services that will pay for themselves and any new infrastructure needed?
When it comes to answering existing questions, diagnostics is an obvious starter. There is plenty of data for an AI algorithm to learn from and there is a shortage of manpower (radiologists, for instance).
Moreover, since most diagnostics are already presented on digital screens, there is usually a convenient way to plug in. So yes, it answers existing questions and provides access to existing systems. With diagnostic trials underway, expect progress and, in time, stunning successes.
What about the third question – those new services?
Clearly, consistent real time diagnostic results every time would take countless days of anxiety away from patients. However, more profound health problems that need addressing include a severe lack of access for patients and increased delays across the system. So, in this context, perhaps we should ask whether AI can revolutionise our capacity to serve?
Answering this will require an entirely different sort of learning. Not just to spot patterns in pictures or combinations of results, but to choreograph the dynamic flows of patients and staff through complicated consultations, interventions and rehabilitation.
There may be a hint of an answer from the world of chess. For years computers have analysed more moves and positions in greater depth than any human, but machines proved uninspiring players and the wins were boring. Positional patterns and relentless analysis, the diagnostics of chess if you like, were not enough.
Then came the legendary AlphaZero, the single system that taught itself chess simply by competing with itself over and over again, and within hours a computer could play like a person, only much better than anyone had played before. This breakthrough came from playing millions of games rapidly, rather than deeply analysing each move.
If we simply feed AI systems with our patient admission and transfer data, we must surely fail to revolutionise care in the way that we need. The learning scenarios evolve too slowly. Plan-Do-Study-Act cycles take many millions of seconds to evolve, which is too slow to exploit the learning potential on offer.
Our experience with the game of chess tells us that we need learning environments where computers can play delivery scenarios. Ones where they can use simple models that they build and run, and rebuild and rerun, all within the same short timescales that are applied in chess.
So, we can see the places where AI is likely to make an early, cost-effective impact in real time diagnostics. To reap the much bigger gain of better structured care delivery, we need to focus not only on the AI, but rather more so on the learning environment. And that means a new focus on healthcare simulation, modelling and digital twins.
Terry Young is Professor Emeritus at Brunel University London and Director of Datchet Consulting.