Coronavirus and the model
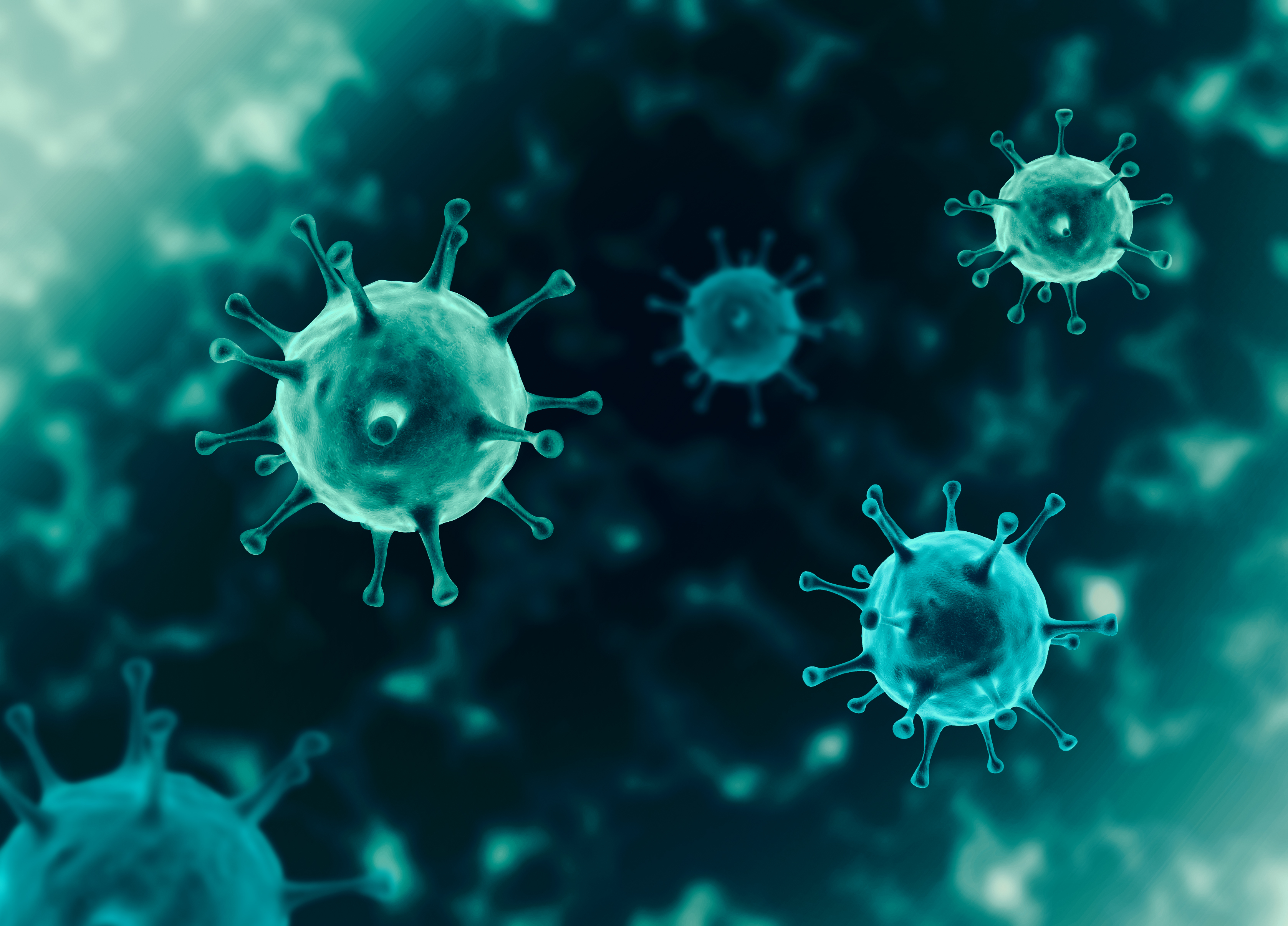
As the Government and its scientific advisers look to publish modelling that has informed UK coronavirus strategy so far, Professor Terry Young, Director of Datchet Consulting, writes about what modelling can offer in this crisis.
The emergence of the model has signalled a new phase in the fight against coronavirus. I haven’t seen it, but my guess is that we are talking about computer code that represents the spread of disease, which is a chain reaction of the sort Diana Ross made famous, although mathematician Tom Lehrer sung a better explanation (I got it from Agnes).
My favourite physicist, Richard Feynman, analysed the radioactive version before computers arrived: if a nucleus goes pop, spraying particles into other nuclei, under what conditions does an explosive avalanche result? Unlike the Manhattan Project, we want to answer the opposite question: how do we avoid the explosion?
Disease models are widely used and underpin plenty of health policy. In areas such as nuclear energy we now know how to slow things down enough to harvest energy safely from the reaction using steam turbines.
So, if the idea of a model is not particularly complicated or new, what is the fuss about?
Two things: the more obvious being what parameters to use. Outcomes can vary enormously even when parameter values change only slightly. For instance, how many people one person will infect. We are not like Feynman’s nuclei which may thought of simply as pre- or post-pop. People may be infectious before they show symptoms, they may experience mild outcomes and recover, and those who recover may be immune to further infection, or not.
When I built models for a living – not of diseases, but of microscopic optical switches and filters, it was easy to simulate new devices. I collected several patents, but it was almost impossible to design products to an exact specification. This was because you needed key parameters to many decimal places, and no two identical devices came out of fabrication.
“Models always trade-off something simple enough to understand against something complex enough to mimic what matters.”
Professor Terry Young, Director of Datchet Consulting
In a similar way, we do not know how transmission rates measured in China or Italy apply here. Modellers usually apply sensitivity analysis and run models thousands or millions of times, changing things slightly with each run. This provides a range of possibilities but says less about what is likely.
We need, then, to learn as we go using our own data. There is a maxim that, ‘all models are wrong, but some are useful.’ Models always trade-off something simple enough to understand against something complex enough to mimic what matters.
The sooner the model is published, the better. This type of fast-learning is difficult, especially since you run with the model, not challenge it (unless or until it proves terminally flawed). By all means, publish as many models as people want to put out there – run competitions! – but the core team will focus on real data and fine tuning.
Clinical models, we get; health logistics models, not so much.
The second problem is more serious because it is invisible to most health policy makers. In the UK, we model diseases and economics well, but we are less familiar with models of logistics and intervention. For instance, models from 20 years ago would have dramatically reshaped today’s urgent care had we heeded them.
Just over 20 years ago, experts used computer models (Lane, et al., 2000) (Wolstenholme, 1999) to show how extra hospital capacity would always fill up, whereas streamlining patient discharge would increase the number treated. Their recommendations were for better solutions at the exit, instead of more capacity at the entrance.
In spite of this, the NHS has continued to invest heavily in extra frontline capacity, so much so that the UK currently has 225 Acute Medical Units (AMUs). While AMUs are an excellent medical idea, they will not ultimately improve patient flow. The overflow problem in urgent care is not primarily clinical or financial, it is about logsitics.
Indeed, coronavirus is a huge logistics problem as well a deadly clinical one. So, let’s think about using the model differently. Is it worth, for instance, trying to identify half of newly infected people within a day, or tracking each infected person’s symptoms, every 12 hours? We can use the model of answer that type of question? If it says, ‘Yes, with the right technology’, we can consider investing in something new.
With our heritage of invention, perhaps we could create a test kit that cost, £1 a pop and gave a good enough result within an hour. The model could also help work out what was ‘good enough’: no test is 100% accurate, and we don’t want too many infected people at large or too many healthy people off work. That type of trade-off is at the heart of decisions now being made.
We have one other asset we could weaponise against the virus: our smart phones. Never before have we been able to track millions of self-isolating people spread across thousands of square miles, but the right app could do that – most of the time. Is that good enough? Use the model!
I don’t know if investing an app-plus-test-kit would be better value than a vaccine just now, but a model could inform a good guess. Maybe we need a quick test to work out who is immune, instead.
So, let’s publish the model, agree not to criticise it, and then use the shared picture to unleash our creative strengths. We have invented tough stuff under tough circumstances for centuries, and this is just another problem we can crack if we can only learn fast enough.
Terry Young is a freelance consultant and Emeritus Professor at Brunel University London. He has been running models one way or another for more than 30 years.