The AI prescription: How generative AI is powering personalised medicine
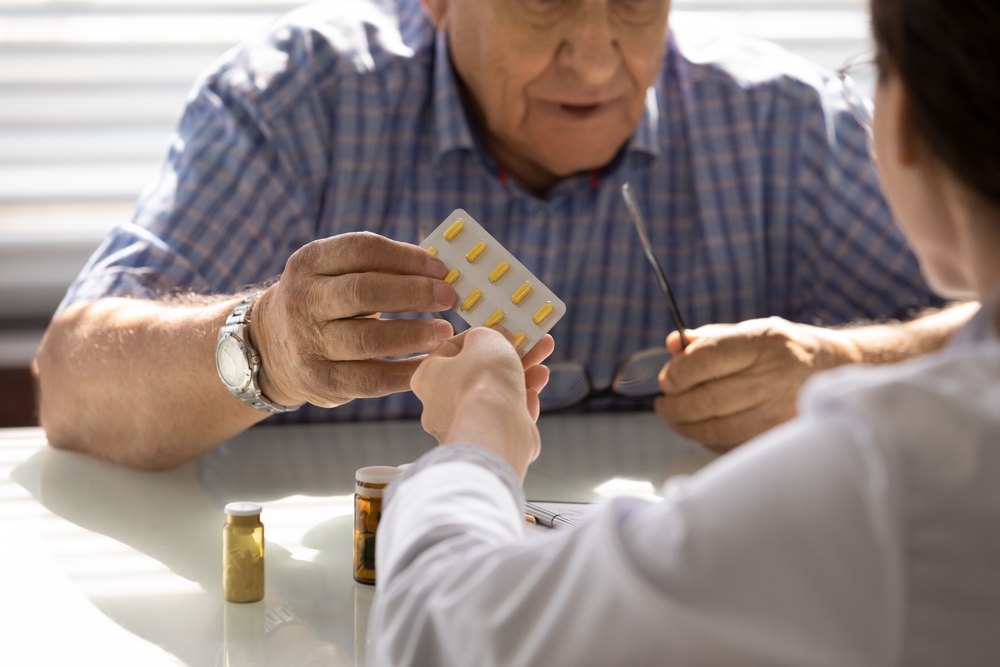
Generative AI is transforming healthcare by enabling personalised treatments, predicting individual drug responses, and accelerating drug development, ushering in a new era of precision medicine, writes Dr Ilya Burkov, Global Head of Healthcare and LifeSciences Growth at Nebius
The future of healthcare is personal. Precision medicine is steering the industry away from blanket treatments and towards tailored care, where decisions are shaped by an individual’s unique genetic code, lifestyle choices, and environmental influences.
Generative AI (GenAI) is accelerating this shift. It can help predict how a patient might respond to a specific drug, flag potential side effects before they occur, and even generate synthetic data to fill gaps in clinical research – ultimately speeding up the development and delivery of personalised therapies.
For instance, two people with the same cancer diagnosis may now follow entirely different care pathways, guided by the specific mutations in their tumours, or how their bodies are likely to metabolise different drugs.
Momentum is strong. A recent McKinsey & Company survey of healthcare leaders across payers, providers, and health tech firms found that 85 per cent were already adopting or actively exploring GenAI capabilities. As the technology matures, it’s on track to become a foundational element of precision medicine.
Understanding individual drug responses
GenAI is enabling better predictions as to how individual patients are likely to respond to specific treatments as well as identifying potentially adverse events before they happen. By training on biomedical data like genomic, transcriptomic, and clinical records, these models can assess a drug’s likely efficacy and toxicity based on a patient’s unique profile.
For example, a study by Wang et al., (2023) leveraged Multi-Omics Integrated Collective Variational Autoencoders (MOICVAE), an AI model capable of accurately predicting drug sensitivity for 25 drugs across seven kinds of cancer. In a separate study, Shi et al., (2023) proposed CSAM-GAN, a generative adversarial network based on sequential channel-spatial attention modules able to predict patient prognosis in lower-grade glioma and kidney renal clear cell carcinoma.
Models like these are helping clinicians match the right therapies to the right patients, while minimising risks through earlier, data-driven insight.
Using AI to go deeper into single-cell data
The rapid growth of single-cell sequencing data is creating new opportunities. So-called single-cell foundation models based on generative pre-trained transformers can distil biological information about genes and cells and be fine-tuned for biomedical tasks, like cell identification, even when data is incomplete. Their broad cellular knowledge makes them highly generalisable across a range of biomedical applications.
Relatedly, GenAI can also be used to create synthetic biomedical training data that closely mimics the statistical variation found in real-world patient data. In the case of rare diseases with few patients, for example, researchers can train models on thousands of AI-generated examples that match the underlying condition to create more powerful and accurate models. Additionally, these datasets can be stripped of personally identifying information and are thus suitable for publication without fear of violating patient confidentiality
Improving outcomes in clinical development
GenAI offers new ways to identify patient subgroups and the relevant biomarkers, crucial for clinical development and maximising drug efficacy. In cancer and other complex diseases, different patients frequently respond differently to the same therapy. Uncovering which biomarkers (or combination of biomarkers) differentiate treatment responders from non-responders is a key part of precision models.
GenAI excels at finding patterns across complex datasets that are hard to analyse using more traditional statistical methods. CSAM-GAN, for instance, is able to integrate a patient’s DNA profile, RNA profile, and histopathology images to predict outcomes — and crucially, pinpoint which biomarkers drive those predictions, whether a gene, pathway, or tissue feature.
Looking ahead, GenAI could even enable the creation of “digital twins” for each patient. Every available treatment plan could then be simulated with the digital twin to identify how each one is likely to play out. While still an emerging concept, this could one day transform personalised medical care.
Faster paths to new medicine
One of the most promising applications of Generative AI is in revolutionising the way new medicines are developed. Traditionally, drug discovery is a long and expensive process, often spanning over a decade and costing billions. GenAI is beginning to upend this model.
By automating key stages and generating viable candidates in silico, researchers can bypass years of trial-and-error. Instead of manually testing thousands of compounds, AI models can suggest those most likely to meet specific targets, for instance, binding to serotonin receptors while avoiding toxicity. This focused approach dramatically reduces early-stage timelines, moving from hypothesis to high-potential drug candidates in a matter of weeks.
Redefining what is possible in personalised medicine
From decoding individual drug responses to creating entirely new datasets and drug candidates, GenAI is rapidly becoming embedded into every stage of the healthcare journey.
The road ahead is even more compelling. Rather than working from general protocols, clinicians could soon draw on AI-powered simulations to test treatments virtually – tailoring care in a way that’s both deeply personal and data-driven. In this new model, healthcare becomes more responsive, proactive, and precise. GenAI isn’t simply enhancing today’s systems; it’s reimagining them, and in doing so, helping deliver smarter, faster, and more human-centred care.
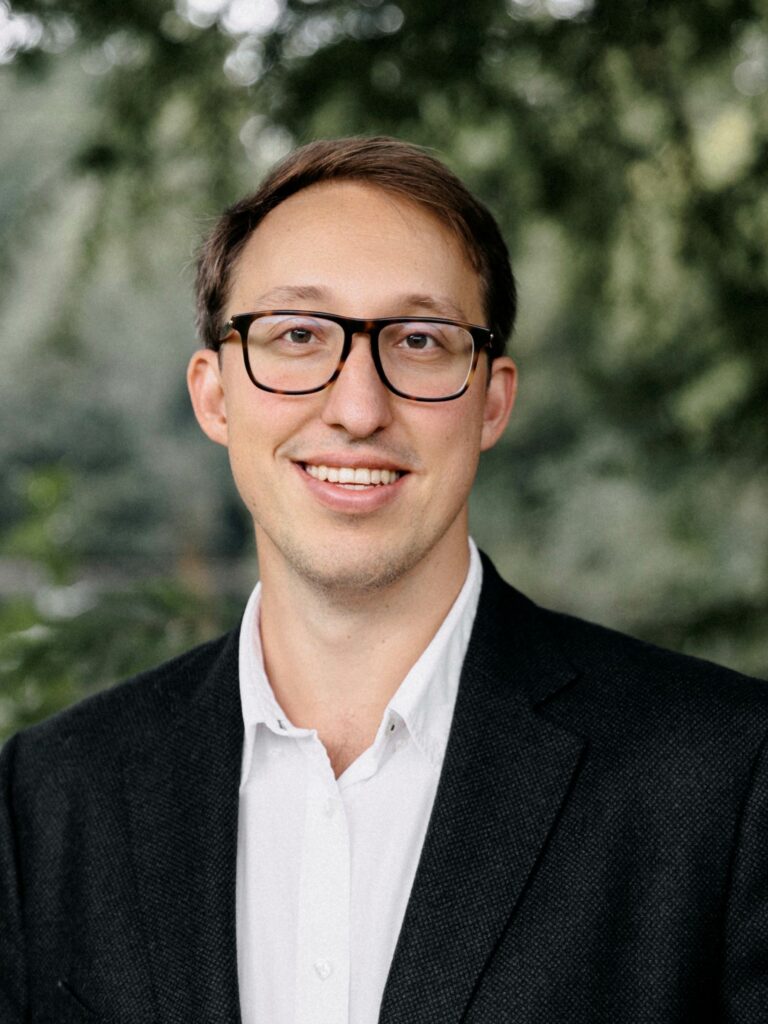