Time to re-think the model?
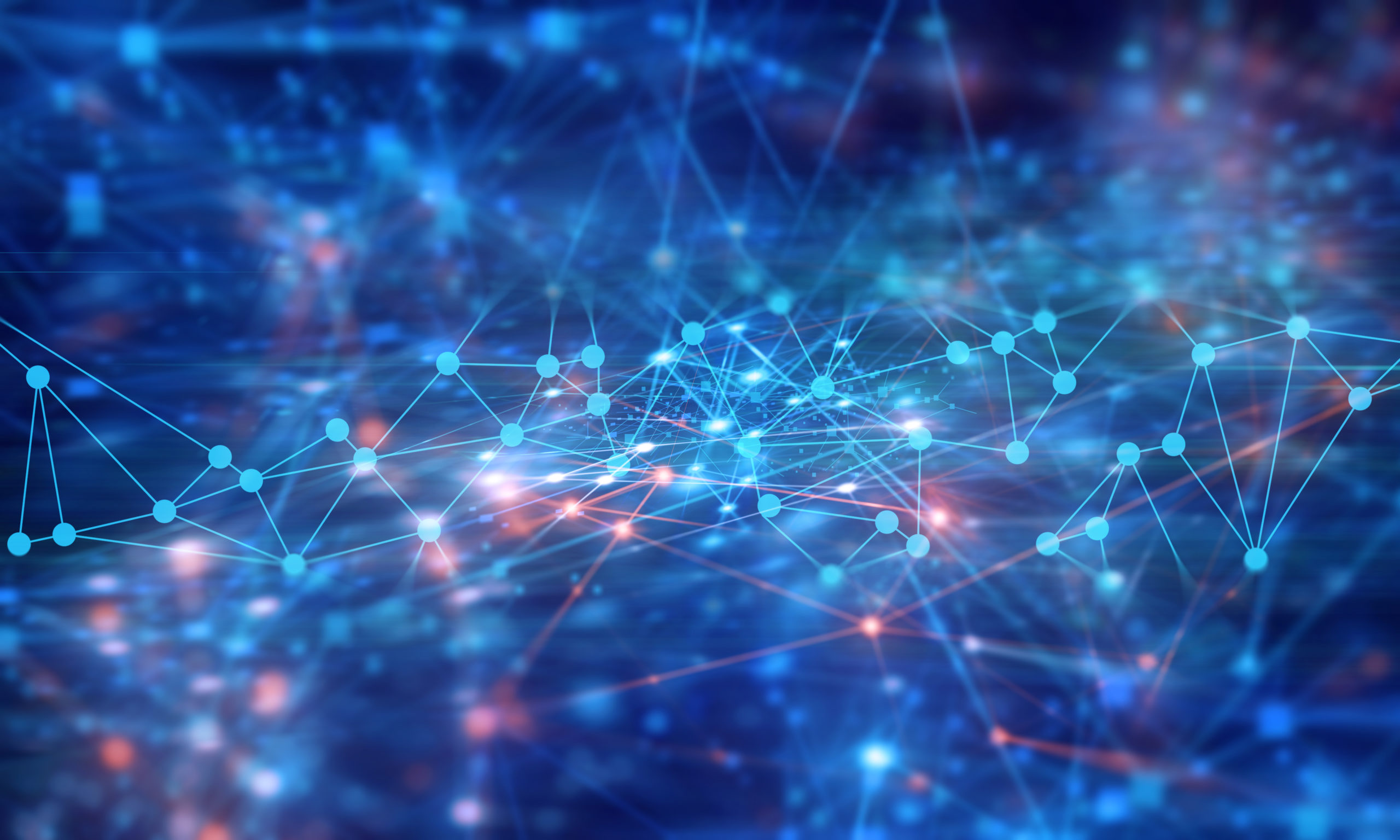
Decisions on which data to use can give very different pictures of the Covid crisis. David Duffy spoke with Professor Karl Friston to outline how linear thinking to data modelling is hampering our response to the pandemic.
Professor Chris Whitty and Sir Patrick Vallance have become household names over the course of the pandemic, and their predictions have had a seismic impact upon our way of life. Their most recent appearance on our screens was to provide ominous warnings of a second wave that could see cases rise to 50,000 a day by the end of October with 200 daily deaths across UK hospitals. Making these predictions requires data modelling – a term that has been thrown around a lot over the past five months, but what do we mean by it?
A data model is an abstract entity that organises each element of a dataset and then standardises how these elements relate to one another. Since the elements of data document real life, the model should represent reality.
The model that is commonly used to measure pandemics by Government and Scientific Advisory Group for Emergencies (SAGE) experts is referred to as an SEIR model – which labels people with four states: “susceptible”, “exposed”, “infected” or “recovered”. This “conventional” method involves taking massive amounts of data from testing results across the country, and explaining these data in terms of how people move among the four states. The computer – usually a super-computer – then calculates what is essentially a “worst–case scenario” based on the current trajectory of the pandemic.
This is a very crude explanation, but it is worth demonstrating that the models used by the Government are limited in their granularity.
“It’s essentially saying, let’s assume that the growth is exponential and then let’s do a press briefing saying what would happen if this trajectory continues,” says Professor Karl Friston, who forms a key component of Independent SAGE, a group of scientists providing Covid-19 advice to the Government and public. Karl is developing his own unique “quantitative” modelling techniques that can potentially provide a more detailed picture of the pandemic.
The trouble with the “conventional” modelling subscribed to by the Government is that with a global pandemic of this scale, the reality does not always allow people to slot neatly into these four categories. “SEIR models start to fall apart when you think about the underlying causes of the data,” says Karl. “You need models that can allow for all possible states and assess which states matter for shaping the pandemic’s trajectory over time.”
The kind of modelling that Karl develops, generative models, are based on his own work at University College London, where he builds mathematical models designed to quantify the transmission of signals between brain areas. When applied to a pandemic – i.e., viral transmission between people and communities – they allow for a much richer and more sensitive prediction. Conventional SEIR-style modelling simply produces a snapshot of the current infection rate whereas generative models can have adaptive Government interventions and surveillance built in. Such as, for instance, a 10pm curfew on pubs, the rule of six or a new contact tracing app.
The accuracy of this modelling in mapping the course of the pandemic has surprised even Karl. When looking back at responses at all levels, the model has been able to predict the profile and shape of the pandemic within a matter of days – and has predicted the course of the mortality burden within a factor of two in most cases.
Karl says that the type of modelling he uses “is what a physicist or an engineer would use, and we can simulate an entire country within seconds on a laptop.” However, he feels as if the “epidemiological orthodoxy” seems to have gone the other way, in favour of stochastic simulations (like modelling a gas by tracking a lot of individual atoms), supercomputers and handwritten C-code. Many of these methods can take hours or even days to generate predictions. “It is a completely different sort of scientific ideology,” he adds.
A more nuanced approach?
This is not to say, of course, that conventional epidemiological modelling is not valid; this is just a different type of – dynamic causal – modelling that, crucially, is able to predict the plausibility of certain outcomes.
It is a different approach, and putting forward different methods is exactly the purpose of Independent SAGE. Karl for one wants to dispel “linear” thinking when it comes to the Covid-19 pandemic and expert opinion around it. “There is an idea that you are either a lockdown denier or a herd-immunity sceptic, and the simplistic nature of current usages of modelling contributes to this.” In his mind, there should be no “adversarial” approach to harnessing measures to fight the pandemic: “all measures against this pandemic, whether it be lockdown or herd immunity, work intimately, hand in hand. They are very delicate but understandable – as long as you have the right model to comprehend them.”
There is an idea that you are either a lockdown denier or a herd-immunity sceptic, and the simplistic nature of current usages of modelling contributes to this
Professor Karl Friston, University College London
For instance, Karl’s model considers that the UK currently has approximately an 8 per cent rate of zero positive herd immunity (based on current antibody testing) – and that this factor works synergistically with Government measures and social distancing adherence.
“Once you get this adversarial premise in play, then any numbers, any source of evidence can be garnered in support of your position relative to somebody else.” This, in Karl’s mind, is where the danger comes in: “you can generate fantastical predictions illustrating what could happen under a certain set of assumptions, and it can go this way or it can go that way. But without any mechanism to evaluate the plausibility of these illustrations, there is very little constraint on what you’re allowed to refer to in order to substantiate your particular position.”
The absence of the ability to score the quality of predictive illustration serves to compound polarisation between experts. The danger is that quantitative, evidence-based approaches are in danger of being overshadowed by more ideological debates. In the face on an increasingly deadly second wave of coronavirus, now more than ever we need collaborative thinking across expert opinion and an honest examination into the strategy that has taken us this far.