Using artificial intelligence to make real improvements to patient safety
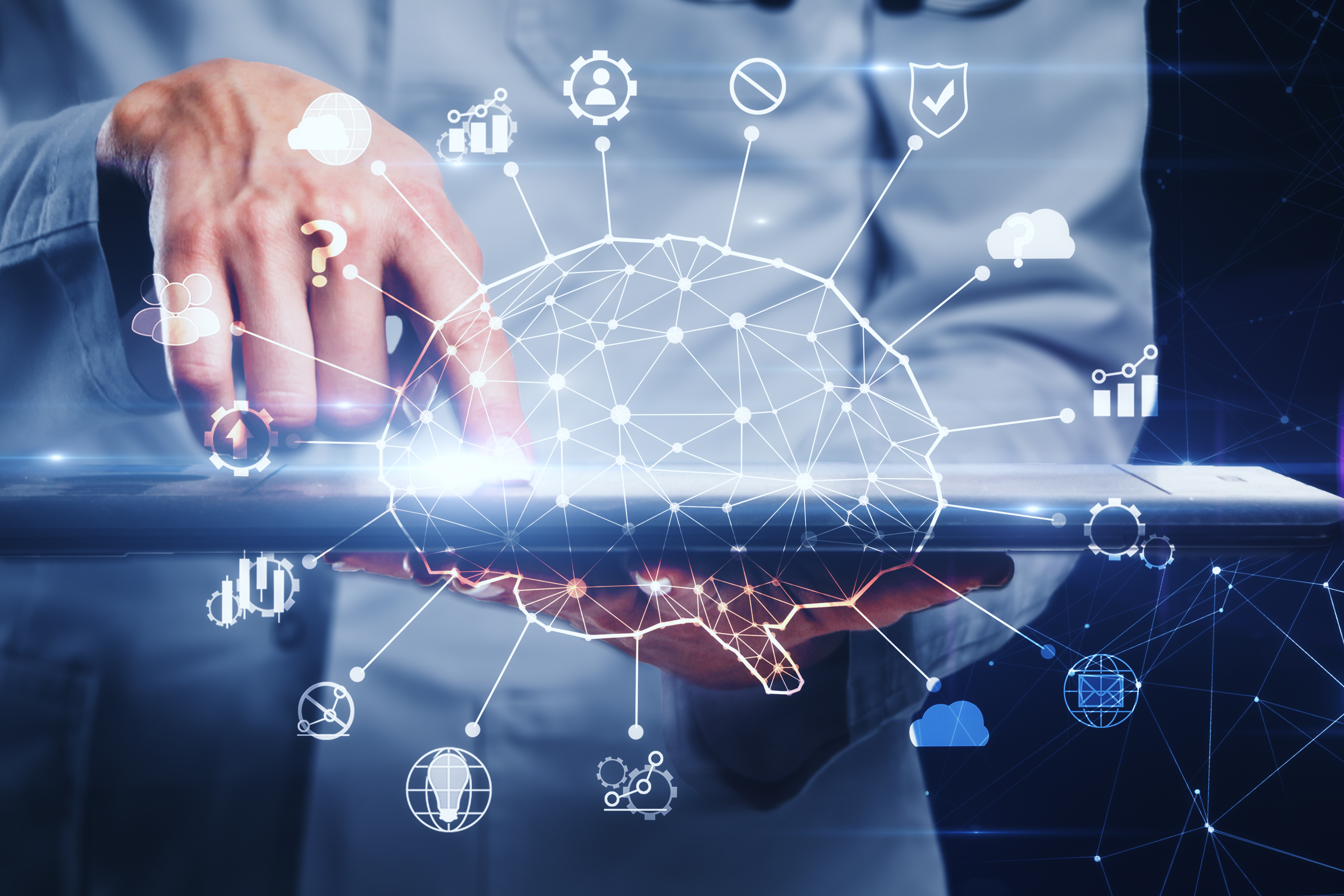
Harness AI to help make sure your hospital is safe, then use it to prove that it is. By Dr Mark Ratnarajah.
“We are on the cusp of a huge health tech revolution that could transform patient experience by making the NHS a truly predictive, preventive and personalised health and care service.”
Those were the words of Matt Hancock, Health Secretary, in August of this year. His comments came as the Government pledged £250 million to boost AI and genomic testing across the NHS. A central aim to this pledge is to make the NHS a world leader in innovation and technology.
Clearly, utilising accurate health data in relation to patient safety will be crucial to realising this vision. The Keogh review in 2013 identified areas of urgent action, as well as some common barriers to delivering high quality care. One barrier identified was the “ability of hospital boards and leaders to use data to drive quality improvement”. The review recognised that this was made more difficult by how hard it is to access data held in different places and different ways across hospitals systems – which is where AI comes into play.
The NHS Board Principles for Good Governance document stresses the need for “evidence-based decision making” in support of a board’s “statutory duty of quality”. Boards are required to endorse and sign off declarations of assurance to regulators in relation to quality. Making sure you are certain of the performance of your safety monitoring systems is vital.
Put simply, clinical AI systems help healthcare institutions reduce avoidable harm, as well as generating significant expenditure savings. Good quality healthcare costs less to deliver (Institute for Health Improvement (IHI) 2003). Increasingly innovative technology allows healthcare organisations to set a benchmark and gold standard for improving quality, efficiency and reducing harm. AI can highlight issues that need resolving and demonstrate, by continual monitoring, that a hospital is safe.
So, when upgrading or implementing a new hospital reporting system, there are several key points to consider.
Identifying past harm quickly and reliably
Some reporting systems produce data 12 months in arrears, whereas others provide a current accurate baseline picture in real-time or after a ‘30 day’ lag. If you’re basing safety and cost efficiencies on this information, immediacy is key to minimise harms and mortality.
Assuming your system has identified an issue, how is this issue then coded and described? Most systems work from Disease Resource Groups (DRG) coding, but DRG codes do not reflect the reality of how clinical departments are organised. Moreover, many systems focus on the first 2-3 diagnostic codes for a patient, but this overlooks how patients will often be referred between a series of doctors – before the correct diagnosis is reached.
Because these systems then aggregate the results into a statistical prediction, they cannot identify which patients may have been affected when generating an alert. So, let’s say you have a received an alert for mortality in ‘bronchitis’; where do you look, which specialty is this happening in, and which cases does it refer to? Your clinicians will understandably pose these questions and will be mistrustful of data that cannot answer them.
However, with the right technology it is possible to accurately identify and code issues in a way which identifies the root cause, and the cases that need to be reviewed. This will then enable clinical teams to resolve issues in a far more effective way.
Learning from mistakes
The Institute for Healthcare Improvement states that a “total systems approach” to patient safety must be implemented to address harm and mortality rates. Identifying avoidable harm allows hospitals to save lives and costs for readmission, admin, medications, bed blocking etc. Making sure reporting also highlights avoidable harms as part of a comprehensive solution within evidence-based safety is crucial.
Improving patient outcomes is only possible if you measure performance accurately. The diagnostic history and risk of each individual patient should be taken into consideration to give a true reflection of both performance and outcomes. If you don’t adjust for risk, then systems will deliver many false positives (e.g. high complication rate alarms that don’t consider the high-risk nature of a set of patients). The result is significant clinical time wasted.
When genuine risk-adjusted methodology is used an accurate picture of performance can be obtained. This can be set against national benchmarks to resolve any underlying issues.
Most reporting systems look at a shortlist of harms that are easy to count, such as pressure ulcers and wound infections, but then produce prodigious amounts of data about them. Or they look at single issues like Acute Kidney Injury – but focusing on identifying and treating rather than preventing and pre-empting. This barely scratches the surface of what is really going on. There are well over 150 traceable complications and harms. These are often interrelated in ways that point to the root cause of a problem that is much further upstream. And in some cases it will be the omission to treat rather than an active error that has led to the problem. It’s only possible to see the whole picture and find the solution if all of these parameters are captured and analysed.
However, it is also the case that the ultimate causes and solutions are simple to report and act upon. The best systems – especially those backed by AI to do the complex groundwork – will not drown you in reams of irrelevant data points, but rather present concise solutions. This will allow more time for making improvements.
Preventing future harm to patients
We must identify patients at greatest risk of harm to ensure they are on the correct treatment pathway. Doing so will allow for greater optimisation of care from the outset, thereby reducing avoidable costs. Can your current system identify those patients at greatest risk of harm, such as acute kidney injury or hospital acquired chest infections before they occur rather than reporting on harm event once they have already occurred?
Dr Mark Ratnarajah is Managing Director (UK), C2-AI – providing unique risk-adjusted systems for individualised patient risk prediction, avoiding harm and mortality in hospitals.